The Future of SaaS Finance: Machine Learning and AI
Explore the future of SaaS finance with machine learning and AI. Learn how to build SaaS financial models with CFO automation tools and optimize FP&A processes.
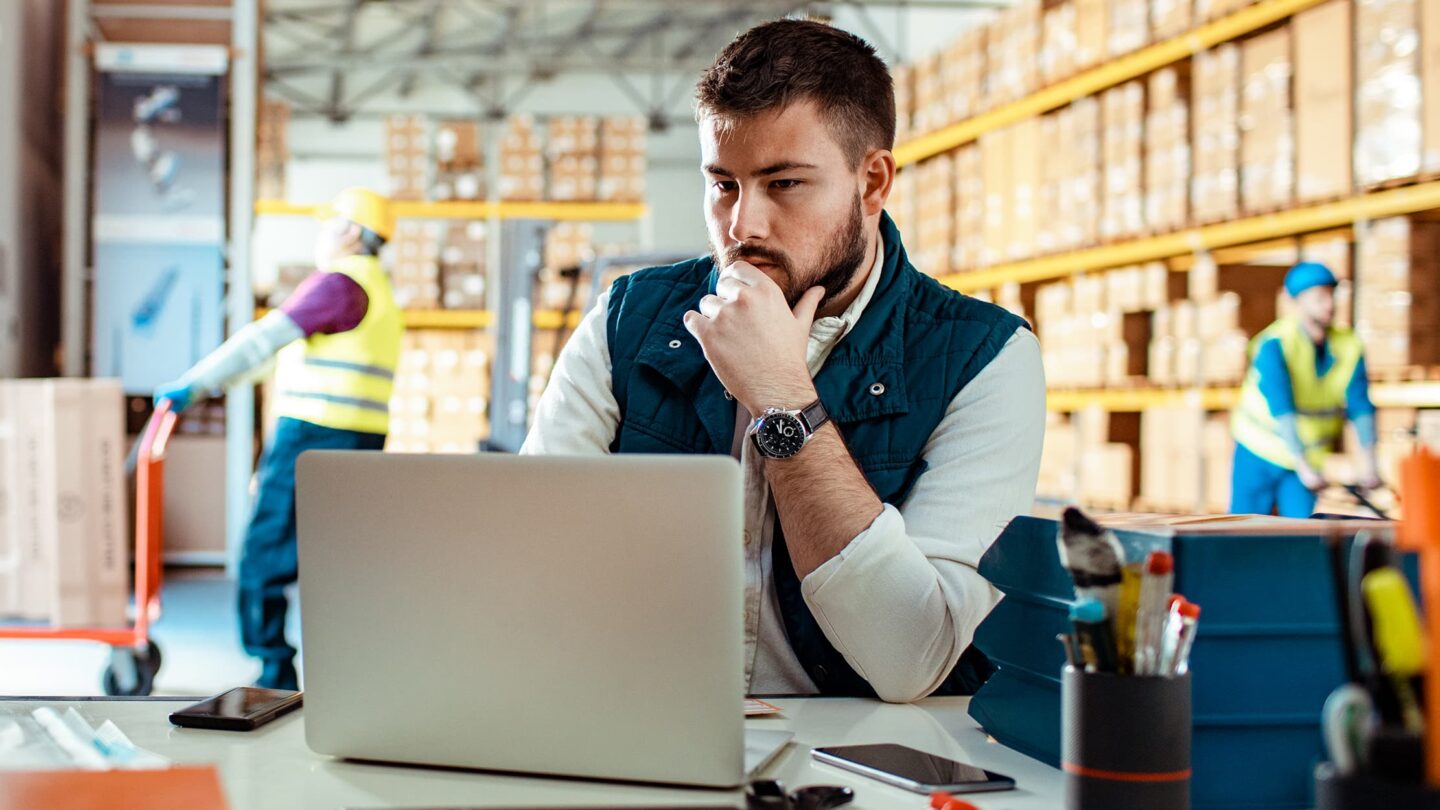
Machine learning and AI will help SaaS CFOs tremendously. How and where? Let’s start with the FP&A function in SaaS organizations that relies on detailed data visualization and financial storytelling. CFO automation tools make those two tasks much simpler and more intuitive for finance teams, among many other benefits.
In this post, we’ll explore the latest trends in financial planning and analysis (FP&A), the benefits and risks of machine learning in FP&A, and how to build a SaaS financial model with machine learning and AI.
What’s next for FP&A?
Financial planning and analysis is the process of budgeting, forecasting, and analyzing financial performance. As SaaS companies continue to grow and mature, they must keep up with the latest FP&A trends to remain competitive.
Some of the top trends include:
- Moving away from spreadsheets and towards dedicated FP&A software
- Automating routine tasks to free up time for analysis and strategic planning
- Focusing on key performance indicators (KPIs) that drive business outcomes
- Using data visualization to communicate financial insights to stakeholders
The challenges facing the FP&A process
Despite these trends, FP&A professionals still face several challenges. Some of the most common include:
- Data silos: Financial data is often stored in different systems and is difficult to access and integrate.
- Manual processes: Many FP&A tasks are still done manually, leading to errors and inefficiencies.
- Lack of automation: Even when software is used, many FP&A tasks are not yet fully automated, leaving room for error and inconsistency.
Automating processes at scale
One way to address these challenges is through automation at scale. Machine learning and AI can help automate routine FP&A tasks, freeing up time for more strategic work.
Machine learning in finance
Machine learning is a subset of AI that uses statistical algorithms to learn from data and make predictions or decisions without being explicitly programmed. In finance, machine learning can be used for tasks such as fraud detection, credit scoring, and forecasting.
Benefits of financial machine learning in FP&A
Financial machine learning enables companies to use algorithms to program software to perform tasks that require human cognition. This has been a game-changer in SaaS FP&A. Some of the top machine learning use cases for SaaS finance include:
- Multiply efficiency: Since it uses algorithmic deep learning, machine learning offloads much of the burden of creating financial models. This increases finance teams’ effectiveness and overall freedom to maneuver.
- Eliminate human error: Using CFO automation tools to create financial models, reports, and forecasts increases the likelihood of human error. This is due not only to errors in the deliverables themselves but from problems with the manual financial processes used to create them.
- Enable rapid data scaling: machine learning enables finance departments to easily work with very large datasets. This makes scaling much smoother and faster by enabling back-end financial processes to keep pace with front-end growth.
When you rely on manual financial processes, you can expect to run into various internal and growth-related bottlenecks. CFO automation tools equipped with machine learning and AI go straight to the source of those problems–manual process reliance–and remove it from the equation.
Machine learning use cases in finance
From presentations to pricing rollouts, machine learning software has a broad range of potential use cases for FP&A teams. Some of the top use cases include:
Flexible and dynamic spending
Machine learning can help companies optimize spending by analyzing data in real-time and adjusting budgets accordingly.
Integrating across daily desktop tools
By integrating machine learning into everyday tools such as email and chat, finance teams can stay on top of financial data without switching between different systems.
Data available across the organization
Machine learning can help break down data silos and make financial data available across the organization.
Automation of processes
By automating routine tasks, machine learning can free up time for more strategic analysis.
Financial visualization in a board meeting
During board meetings and presentations, financial visualization with financial machine learning software can help your audience easily make sense of complex ideas and relationships.
Building flexibility into your campaigns
One of the major benefits of algorithmic FP&A is the ease and simplicity of altering your financial models. Compared to manual accounting methods, this leads to higher accuracy and lets teams quickly compare and contrast small adjustments.
Process unification across departments
Siloed data and disconnected processes scattered across departments can lead to serious operational liabilities over time. Financial machine learning software uses the cloud to sync data updates and operations across departments.
This has a positive impact on revenue recognition and many other financial processes.
As appealing as it is, however, machine learning for SaaS finance isn’t necessarily foolproof or without its limitations. Let’s examine a few of them.
Risks and limitations of financial machine learning in FP&A
Despite the above benefits, there are also risks and limitations to using machine learning in FP&A. Some potential hurdles to be mindful of include:
- Lack of data: If you’re a brand new company or you’ve only recently launched, you might not have sufficient data to justify an investment in machine learning. Remember, machine learning models must be trained on your specific datasets before they’re of any use to you personally.
- Bias in the data: Biased data refers to data that’s unbalanced and therefore difficult for machine learning to accurately work with. You might get “workable” models from biased data, but they won’t be accurate. If your company only recently launched, your dataset might be overbalanced to reflect an initial adoption rush of one type of customer.
- Inability to blend with legacy tech: Unlike cloud-enabled accounting tools, machine learning applications are incapable of “lifting and shifting” onto legacy accounting tech. This requires a firmer commitment to moving away from older tools before you make the machine learning leap.
- Model continuity problems: Often, only one or two people on a finance team fully understand how to craft and manipulate machine learning models. That can be a considerable liability if they leave the company.
With all this in mind, how would you ideally get started with AI and machine learning in your SaaS finance department?
Getting started with machine learning and AI
Now that we’ve covered the benefits and risks of machine learning in FP&A, let’s dive into how to build a SaaS financial model with machine learning and AI. When you’re first getting started with AI and machine learning, keep these four important best practices in mind:
1. Make sure everyone in your department–as well as your other stakeholders–understands why the shift matters and what will be gained.
2. Take a second to double-check that you won’t be stuck relying on outdated tech that will complicate or slow down implementation.
3. Talk with the other leaders at your firm and get ideas and thoughts on clear FP&A goals and results that people would like to see. What’s their mental image of your company’s FP&A before and after implementing machine learning and AI?
4. Continue to check back with stakeholders and department members as the rollout unfolds, and periodically afterward as well. How are they feeling about the recent change? Have any of their stated goals around machine learning and AI been met?
Let’s dive back into specific benefits and use cases of FP&A advanced analytics machine learning for SaaS accounting teams.

Financial machine learning for SaaS FP&A
Financial analysis relies on quickly and accurately working with large datasets that are split into many different categories. CFO automation tools make it considerably simpler to scale, manage, and use your data.
Finance pros use FP&A advanced analytics machine learning to help companies chart their present and future cash flow. This helps organizations:
- Make effective hiring calls: Because effective hiring often involves multiple layers of “if-then” forecasting, FP&A advanced analytics machine learning can be very helpful. Built-in model flexibility makes it easier to forecast potential scenarios, and the elimination of manual forecast assembly reduces variance significantly.
- Successfully navigate recessions: One of the biggest benefits of AI and machine learning is the clarity they bring to recession FP&A. Accounting software equipped with AI and machine learning allows finance teams to manage their cash flow in a much more granular and effective way than legacy systems are capable of. When the markets head south, this is invaluable.

- Cut operational costs with automation: Financial process automation enables finance teams to maximize their budgets by relegating repetitive but essential tasks to software instead of humans. Rather than eliminating the need for employees, this frees them up to make more valuable contributions.
Let’s look at machine learning as it relates to financial models.
Building models with financial machine learning?
As the name implies, machine learning enables software applications to autonomously learn to create predictive models. This is the backbone of FP&A advanced analytics machine learning.
Let’s compare legacy financial modeling to financial modeling done with CFO automation tools. With legacy accounting systems, financial modeling has two primary steps–speaking very broadly of course.
Step one is to gather and organize large sums of financial data, customer data, and other types of info relevant to FP&A. (Machine learning and AI simplify and streamline this otherwise complex and lengthy task.)
Step two is to manually organize this data, and then use tedious spreadsheet formulas or a similar method to assemble reports and forecasts.
When accounting teams invest in FP&A advanced analytics machine learning, step two is taken care of automatically. CFO automation tools equipped with machine learning can autonomously use existing data pools to create forecast models, budget models, and pricing models, among other varieties.
What is the use of machine learning in forecasting?
FP&A advanced analytics machine learning gives CFOs more confidence in the various forecast models they use.
Machine learning plays an important role in SaaS FP&A forecasting by:
- Considerably reducing forecast variance.
- Enabling more complicated multifactor forecasts.
- Significantly extending forecasts’ effective timeframe.
- Allowing teams to alter financial projections with a single click.
FP&A advanced analytics machine learning simplifies and streamlines SaaS FP&A for modern accounting teams. CFO automation tools make it easier for SaaS finance professionals to get an accurate read on a company’s financial future.

See what the future holds
SaaS finance is changing at a more rapid pace than ever before. AI and ML are causing teams to think about workflows and employee roles in a new light. Advances in accounting technology are allowing finance leaders to work much more effectively, but are also making the landscape much more competitive.
That’s why we created the Modern SaaS Finance Academy, a collection of online courses taught by industry leaders and experts designed to help SaaS finance pros level up their FP&A results, cut down on forecast variance, and much more. Each curated lesson helps finance and accounting leaders learn the skills and perspectives to scale the business to IPO and beyond.
Click here to join the academy today.
Modern SaaS Finance Academy
The Modern SaaS Finance Academy is a free online training hub designed for CFOs, Controllers, FP&A, Revenue managers, Revenue Operations, and other members of the finance community in fast growth SaaS companies.
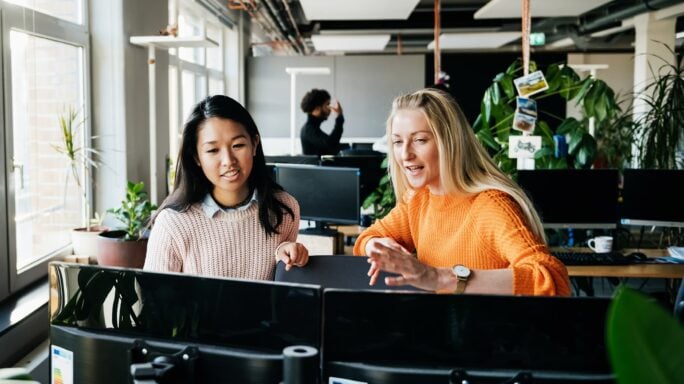
Ask the author a question or share your advice